- Developers
- Developer Blog
- FinTech Software Development
- How to Use Big Data for Finance Industry?
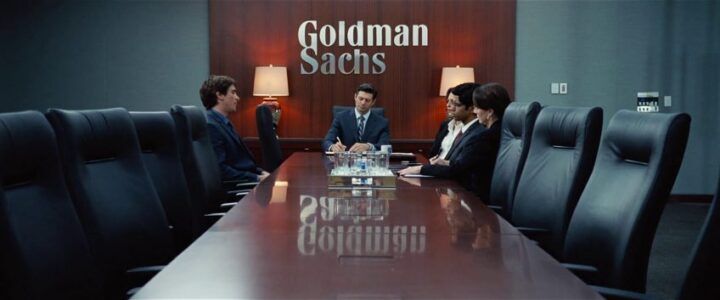
profile

By Sam Palmer
Verified Expert
1 year of experience
Sam is a professional writer with a focus on software and project management. He has been writing on software-related topics and building PHP based websites for the past 12 years.
This blog discusses how businesses can use big data for the finance industry.
Big Data for Finance Industry
Banks and insurance companies used to basically guess which people would likely pay back a mortgage on time, or wouldn’t accidentally burn their house down. These days, they rely on this new ‘Big Data’ to help them make better guesses.

Big data analytics involves collecting and analyzing this new wave of information. It can be historical data stored in a database somewhere, or information being collected right now by a bank, shopping center, or insurance company.
Analyzing large amounts of data can happen in many different ways. Complex systems and algorithms are built especially for this purpose, and every financial institution uses them.
The big data is analyzed for patterns that can be used to predict future trends, calculate risks, or to determine prices.

Which Financial Services Use Big Data Analytics?
The main financial services are:
- Credit unions;
- Banks;
- Credit card companies;
- Insurance companies;
- Accountancy companies;
- Consumer finance companies;
- Stock brokerages.
These days, all of them use data analytics, and most of them are completely dependent on it to function. Financial institutions like banks, credit card companies, and credit unions use historical information to determine the risk level of borrowers.
The financial services sector set interest rates based on these figures. Insurance companies use all sorts of unstructured and structured data to determine the risk of just about anything and set premiums accordingly.
Hire expert developers for your next project
Let’s take a look at some examples of how financial services firms are leveraging big data technology right now.
How Is Financial Industry Using It?
There are many ways financial companies can go through big data adoption to improve their products, reduce costs, and make customers happy.
1. Improve Customer Segmentation
Banks can use customer purchasing data to find out which products, mortgages, or credit cards different types of people are likely to buy. They use this information to segment customers into groups with similar financial situations and goals – and laser-target their sales and advertising.
That means customers get more recommendations for products they probably want, and banks spend less time trying to sell to people who probably aren’t going to buy.
2. Developing New Products
That idea can be taken even further and used to develop new products that will sell. Combining customer valuable data with economic trends can help predict what financial products will be popular this year, or next. Banks and insurance companies can develop and market the right products at the right time.
Consumers get more products that suit their needs, and banks waste less money and earn more profit.
3. Detecting Fraudulent Activities
Modern cyber threats to big companies are often extremely sophisticated and can cause serious damage. Every IT system has vulnerabilities, and security breaches can often go unnoticed for months. The sheer scale of IT systems now means that manual monitoring for threats just isn’t feasible.
Data analytics is the new tool security engineers are using for fraud detection.
For example, a bank can monitor customer spending patterns to detect anything abnormal in real-time financial transactions. If a usually conservative spender suddenly starts taking out loans and going on shopping sprees, big red flags will go off.
This is a powerful tool to stop fraudsters and thieves in their tracks.
Hire expert developers for your next project
1,200 top developers
us since 2016
This helps keep theft and damage to a minimum, which keeps costs and prices down for customers.
4. Lowering Investment Risks
All financial companies earn a profit by making good investments. This could be making loans to people who’ll pay them back, or selling car insurance to someone who is a safe driver. This used to be the work of experts who would make an educated guess as to which investments would likely pay off.
Nowadays, financial companies analyze huge databases of information to find out which investments will likely pay off, and which ones won’t. And they do it with surprising accuracy.
Banks use this to calculate the risk of different homebuyers and set interest rates. Insurance companies do the same with home insurance premiums.
5. Sentiment Analysis
One way that social media data is leveraged by stock brokers is with sentiment analysis – or opinion mining. This means using clever natural language processing algorithms to figure out what people really think based on what they say.
These predictive analytics techniques are used to analyze millions of tweets and Facebook comments to guess what is happening in the world right now and react instantly.
Many companies use this to build trading algorithms that can quickly bet against stock prices immediately after a disaster hits. If you are the first to understand that a plane has crashed, you can quickly bet that Boeing shares will drop, and earn a huge profit.
You only need to be milliseconds faster than your competition for this to work.
What This Means for You?
The domain of big data analysis is still developing, and the next few years are going to have successes and disasters. You can expect your financial services to get better and smarter quickly as big companies have better tools and their financial market is facing more competition.
Read how you can use artificial intelligence to improve your fintech app on our blog here.
Hire expert developers for your next project
However, in the race to be the best, some companies are going to make mistakes. This will come at a big cost to them and their customer satisfaction, so make sure you’re careful about who has your market data!
If you are also planning to use big data analytics for finance business models, make sure to partner with a professional and trustworthy team of software developers and data scientists.
Data science and machine learning are domains of complex technical skills. You will need exceptional data engineers to successfully complete your data analytics for a finance project.
DevTeam.Space can help you here. We have a community of field-expert software engineers who are experienced in hybrid software development using cutting-edge technologies.
You can write to us your initial requirements for data analytics for finance solutions through this quick form. One of our technical managers will get back to you for further assistance.
Frequently Asked Questions on Big Data Analytics for Finance

It is the use of computer programs to analyze and interpret large pools of structured and unstructured data.
Big data is being used extensively in the financial services industry for everything from identifying fraud, and risk management to helping to improve the accuracy of trading bots using internal and external data sources. Read the above article for more information.
Big data analytics has the power to analyze huge pools of data that would take large numbers of humans months. This means that trends or patterns can be quickly identified and acted upon. Along with this, big data analytics programs can also analyze data pools that were previously too large or deemed too complex to analyze.
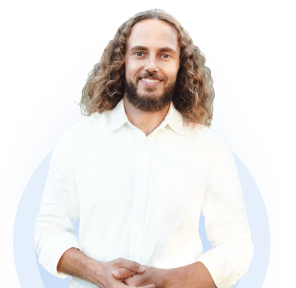
Alexey Semeney
Founder of DevTeam.Space
Hire Alexey and His Team
To Build a Great Product
Alexey is the founder of DevTeam.Space. He is award nominee among TOP 26 mentors of FI's 'Global Startup Mentor Awards'.
Hire Expert Developers