- Developers
- Developer Blog
- AI Software Development
- What is the Future of Machine Learning in Software Development?
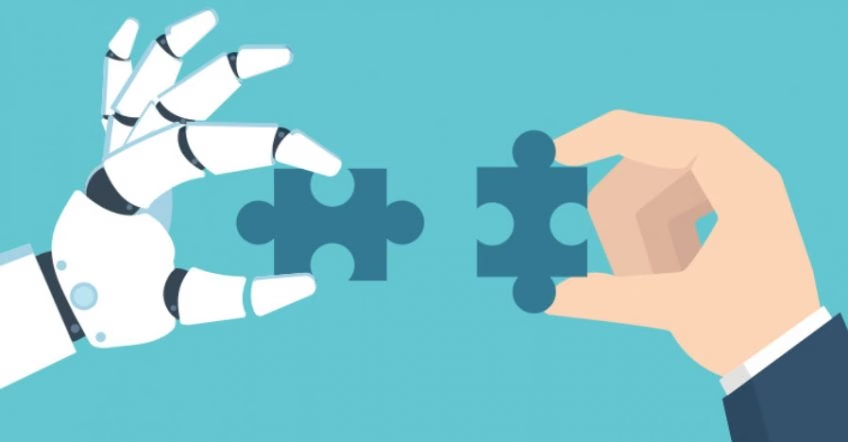
profile

By Aran Davies
Verified Expert
8 years of experience
Aran Davies is a full-stack software development engineer and tech writer with experience in Web and Mobile technologies. He is a tech nomad and has seen it all.
In this blog post, we are discussing how machine learning in software development is going to look like in the future for businesses across various industries.
ML can bring several positive changes to software development, and I will now explain them.
1. Detect deviations from coding guidelines
Well-written software must meet its functional and non-functional requirements, moreover, it should also follow relevant coding guidelines. Programmers that follow coding guidelines consistently write code that avoids unnecessary complexities, furthermore, such code is easy to understand.
You can read more about the importance of coding guidelines in “Importance of code quality and coding standard in web application, mobile app and software development”.
How do you ensure that your team follows coding standards? Well, code review is the only way, however, that‘s an expensive affair! You need to ensure that reviewers focus on what matters, therefore, you need tools that can find common deviations from coding standards in software engineering.
Machine learning can help here since ML-powered tools can find such common deviations. This can have a significant positive impact on your software development projects since coding standard deviations give rise to many application security risks, as the Open Web Application Security Project (OWASP) Top 10 Application Security Risks – report highlights.
2. Obtain insights from code
If you are a senior leader in an enterprise IT division, you know how complex it can get! Various factors create a complex environment in most enterprise IT departments, e.g.:
- There are far too many projects with conflicting priorities.
- Different vendors have sold products that address the same functionalities, and now you have too many silos!
- Different business leaders set up their communication channels with the IT team and sponsor their projects, which stretches the thin IT capacity.
- The IT department is tasked with maintaining the existing systems, moreover, the same team is often tasked with new development. This creates conflicting priorities.
- A typical enterprise IT division has many external IT consultants, and it‘s hard to ensure that their work aligns with the enterprise strategy.
- You find it hard to get a reliable view of the capabilities and skills of your IT team.
- Different IT managers prefer different frameworks and tools, and now you have a huge portfolio of them, without knowing whether all of them are useful!
Read “Here‘s why enterprise IT is so complex” to learn more about the complexities in an enterprise IT organization.
Get a complimentary discovery call and a free ballpark estimate for your project
Trusted by 100x of startups and companies like
You need to simplify this, and that will likely be an important transformation. However, you need insights even to plan such a project and ML can help here.
You can run an ML-powered tool to study your code on repositories like GitHub for data analysis, and gain actionable insights. An example of such an ML-powered tool is source{d}. It can provide several key insights, e.g.:
- What is the extent of legacy code in your IT portfolio?
- Do you have code that isn‘t maintained?
- How many apps do you have that aren‘t adapted to the cloud?
- What % of your apps isn‘t containerized?
- What slows down your development process?
- How often do you reuse code in your organization?
- Who are your top-performing programmers?
- How effectively does your team collaborate?
- What key skills do you lack in your team?
3. Simplify software development project management with Machine Learning
Managing a software development project can be complex in an enterprise IT context. Software development project managers (PMs) need to contend with complexities in several tasks, e.g.:
- Schedule and cost estimation;
- Tracking project status and costs;
- Managing quality;
- Risk management;
- Human resource management.
You can read more about this complexity in “Software engineering | software project management complexities”.
ML-powered PM tools can help PMs to navigate this complexity. Easy Projects is a good example of a provider offering such capabilities, and its solution offers the following features:
- Easy Projects uses Machine Learning and Artificial Intelligence for project forecasting, Machine Learning algorithms can help PMs to forecast when the project will be completed.
- These algorithms factor in several variables like the project team composition, the past performance of the team members, the rate at which the team completes its tasks, etc.
You can read about this solution in “Machine learning project forecasting”.
ML-powered tools can use organizational information repositories and external data to help the PM to identify risks. You can read more about this in “Traditional vs machine learning for software development paradigms”.
PMs can use ML-powered tools to create network diagrams, work breakdown structures (WBSs), etc. Such tools can expedite the review of key project documentation, and they can help with project status tracking.
We at DevTeam.Space use data-driven processes including AI and ML-powered real-time dashboards.
4. Make coding, code review, and testing easier with Machine Learning
As a senior leader in an enterprise IT division, you know that there are plenty of manual, repetitive tasks in application development, code review, and testing. Well, machine learning is now bringing a whole new wave of automation that is well beyond the rule-based automation that you have seen earlier.
Hire expert developers for your next project
1,200 top developers
us since 2016
Let‘s review a few examples:
Stack Overflow Autocomplete
Emil Schutte, a San Francisco, California, USA-based software development expert has created Stack Overflow Autocomplete, which can reduce coding effort. This ML-powered tool currently works for JavaScript development only.
This tool is far more than simple rule-based automation since it can understand the functionalities offered by code in Stack Overflow. It factors in the intended functionality and creates new code from what it has “learned” from Stack Overflow.
DeepCode
We all know that code review involves plenty of manual effort. If a tool can diligently find serious coding errors, then it can help experienced reviewers. DeepCode goes beyond traditional code review tools since it “learns” from source code repositories to find critical bugs in the code.
DeepCode works with Java, JavaScript, and Python programming languages. Its machine learning algorithms “learn” from thousands of high-quality open-source repositories. This tool can analyze the intent of the code. You can use DeepCode on the cloud, alternatively, you can install it on-premise.
Let‘s take an example to understand how this goes well beyond simple rule-based automation programs. As you know, finding crucial security vulnerabilities like cross-site scripting (XSS) and SQL injection can be hard with traditional code review tools. DeepCode can find these types of vulnerabilities, and that‘s a very important capability.
applitools
You know visual testing and monitoring requires a significant manual effort, e.g., you need to configure various parameters in the testing framework. Your team needs to modify visual processing settings, and they need to do this for multiple platforms.
With applitools, an ML-powered testing tool, it‘s very different! ML-algorithms are adaptive, therefore, you don‘t need to do those manual configurations. With this visual UI testing and monitoring tool, you can find potential bugs without explicitly specifying elements.
Applitools isn‘t the only such machine learning testing tools. You can find more such examples in “8 innovative AI test automation tools for the future: the third wave”.
5. Manage Code Deployment and Production Environment
Software code maintenance is an on-going task. There are new versions to be tested in an already running production environment. Machine learning algorithm help in version control of code base without causing disruptions in deployment phase. Neural networks are able to learn trends from the data and alert maintenance engineers of the potential fault beforehand.
Hire expert developers for your next project
6. Improve Data Management with Machine Learning
Machine learning models work effectively on big data where they are able to learn broader range of patterns and trends. Ensuring faster response time and lower memory consumption automatically becomes complex tasks for data science professionals.
Machine learning make data integration from multiple sources way easier then traditional data indexing. Moreover, machine learning helps with the management of data infrastructure, helping data engineers to manage data piplelines efficiently.
Planning to transform software development in your organization with the help of machine learning?
There are various ways in which machine learning can change software development in your organization, however, it can be hard to build such ML-powered solutions. Machine learning is a niche skill, and building such a solution is a complex project.
I recommend that you engage a reputed software development company for such projects, and read our guide “How to find the best software development company?” to find one.
If you are still looking for experienced artificial intelligence developers and data scientist to create software products for your next business idea, DevTeam.Space can help you. Write to us your initial machine learning software development requirements via this quick form.
One of our technical managers will get back to you to assist you further while linking you with software engineers and data scientists with relevant industry experience.
Frequently Asked Questions
Machine learning involves using algorithms to allow computer software programs to ‘learn’ different tasks making use of the available data. ML programs become more accurate the more they train on the relevant test data.
AI stands for artificial intelligence and aims to mimic human brain activity in the form of an artificial neural network. ML for machine learning. Current solutions are really ML as they are limited in the new things they can learn and lack any ability to understand emotions, etc. Machine learning is considered as a subset of Artificial Intelligence.
The most sophisticated example of machine learning at the current time is the chatbot. Chatbots are able to learn complex patterns of speech as well as accents, etc using different machine learning approaches like natural language processing, deep learning, etc.
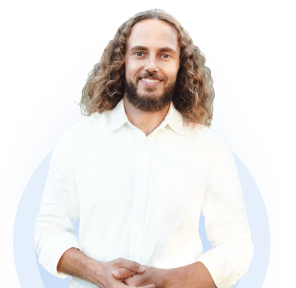
Alexey Semeney
Founder of DevTeam.Space
Hire Alexey and His Team To Build a Great Product
Alexey is the founder of DevTeam.Space. He is award nominee among TOP 26 mentors of FI's 'Global Startup Mentor Awards'.
Alexey is Expert Startup Review Panel member and advices the oldest angel investment group in Silicon Valley on products investment deals.