- Developers
- Developer Blog
- AI Software Development
- Top 10 Machine Learning Algorithms 2024
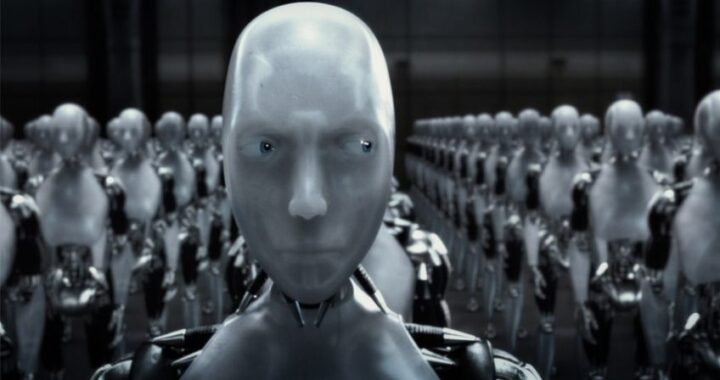
profile

Verified Expert
1 year of experience
Alexey Semeney is an entrepreneur, founder, and CEO of DevTeam.Space. He is a product development expert and an occasional writer on different topics related to team management and product development. He is an avid traveler and sports enthusiast.
The following are the top 10 machine learning algorithms examples based on popularity and real-world usage.
Artificial Neural Networks
Artificial Neural Networks are named so because they’re based on the structure and functions of real biological neural networks. Information flows through the network and in response, the neural network changes based on the input and output. This machine-learning algorithm is used in a number of ways:
- Character recognition (understanding human handwriting and converting it to text)
- Image compression
- Stock market prediction
- Loan applications
One of the most common uses for Artificial Neural Networks is speech recognition. If you’ve ever used Siri, you’ve probably used an ANN. These types of machine learning algorithms get better with more information – they’re constantly growing. Let’s be real: speech recognition has grown leaps and bounds in accuracy over the last five years.
Naïve Bayes Classifier Algorithm
The Naïve Bayes Classifier Algorithm is a classification machine learning algorithm that works off of the popular Bayes Theorem of Probability. It’s one of the most popular learning algorithms that groups similarities, and is usually used in the following ways:
- Disease prediction
- Document classification
The Naïve Bayes Classifier may sound unfamiliar, but you’ve probably encountered it before. The most popular examples are your email spam filter and RSS feeds that filter news into specific categories (Politics, Entertainment, Sports, etc.).
This algorithm is particularly useful if you have a moderate or large dataset, if the new data has several attributes that can help classify it and if the attributes that describe a certain classification are conditionally independent.
Support Vector Machine Learning Algorithm
Support Vector Machine is one of the many examples of machine learning algorithms catered to classification. This is used for either classification or regression in instances where the set of data teaches the algorithm about specific classes so it can classify newly added data. SVM is constantly growing and evolving.
Hire expert developers for your next project
SVM is commonly used in:
- Stock Market forecasting
- Risk assessment
Most commonly, SVM is used to compare the performance of a stock with other stocks in the same sector. This helps companies make decisions about where they want to invest.
K-Means Clustering Algorithm
The K-Means Clustering Algorithm is one of the most popular machine learning examples. It is commonly used in the following applications:
- Search engines like Yahoo and Bing (to identify relevant results)
- Data libraries
- Google image search
- Microsoft Machine Learning Studio
K-Means Clustering is a simple machine learning algorithm used for clustering, meaning it helps group together similar data sets. This could be anything from images and videos to text documents and web pages. For example, you’re searching Wikipedia for the word Apple.
This could bring up results for both Apple, the technology company, and apple, the fruit. K-Means Clustering would group together results about the technology company apart from results about the fruit, so you can get meaningful results on the actual topic you want to read about.
K-Nearest Neighbors Algorithm
Like K-Means Clustering, K-Nearest Neighbors is another classification and regression machine learning algorithm. It’s most commonly used in:
- Pattern recognition (like to predict how cancer may spread)
- Statistical estimation (like to predict if someone may default on a loan)
K-Nearest Neighbors makes predictions by searching through the whole dataset to find the most similar instances (the neighbors) and summarizing the output variable for those instances. Objects are classified by majority votes and assigned the class most common to its neighbors.
Decision Tree Machine Learning Algorithm
Decision Trees are graphical representations that show all possible outcomes of a decision based on certain conditions. It’s typically used for two things – to classify or to predict – and remains one of the best machine learning algorithms for classification. It has been used in the automate the following functionalities:
Hire expert developers for your next project
1,200 top developers
us since 2016
- To help banks classify loan applicants and their probability of defaulting payments
- To help Gerber Products decide whether or not to use PVC in their baby products
- Identify at-risk patients and disease trends with Guardian, a tool developed by Rush University Medical Centre
The decision tree algorithm falls into either Classification Trees or Regression Trees. Classification trees are the default and used to split data into different classes based on the response variable. Regression Trees are used when the target variable is continuous or numerical. This is typically used in a predictive nature. Because of the nature of the algorithm, if your data has errors, so will your decision tree. These classification problems mena it’s best suited to extensive, meticulously correct data.
Apriori Machine Learning Algorithm
Apriori algorithm is a data-mining machine learning algorithm that generates association rules for a given set of data. It has been used in everything from a college elective system that helps students choose classes to a database that discovers the social status of diabetic people. Its most popular applications include:
- Google auto-complete
- Amazon and AWS shopping recommendations
- Detecting adverse drug reactions
- Natural language processing for chatbots
Apriori works by using association rules from a given data set. These rules imply that if A occurs, B also occurs. For example, Wal-Mart actually used the Apriori algorithm to increase sales of beer. Wal-Mart studied their big data pools to find that American males who bought diapers on Friday afternoons also frequently bought beer. They moved the beer next to the diapers, and sales increased.
Apriori is beneficial in more than a couple of ways. It also happens to be one of the easiest machine learning algorithms to implement.
Linear Regression Machine Learning Algorithm
Linear Regression is one of the most interpretable machine learning algorithms. It’s easy to explain to others and requires minimal tuning. This is perhaps why it’s one of the most popular algorithms. It can be used to:
- Estimate Sales
- Assess Risk
Linear regression works by showing a relationship between two variables and how the change of one variable affects the other. This is why it’s so great in risk assessment and business.
For example, health insurance brokers often use this algorithm to allow for automation of analysis of the number of claims per customer against their age, etc. If insurance companies find that older customers tended to make more claims, they increase rates for older customers. If they found that older customers didn’t have more accidents, they could lower the rates.
Random Forest Machine Learning Algorithm
Random Forests or Random Decision Forests are a machine learning method of classification and regression. You’ve probably seen them used in the following ways:
- To help banks predict high-risk loan applicants
- To predict failure or breakdown of a mechanical part
- To predict if a patient is likely to develop a chronic disease
- To predict the average number of social media shares on a post
Its versatility is what gives this algorithm its popularity. Instead of using a single decision tree, Random Forest uses a multitude of decision trees to come up with a solid classification or prediction.
This ensures a more accurate classification because each decision tree is given slightly different data. These variables are very effective because they help preserve accuracy when data is missing. It’s also fairly resistant to outliers (majority always rules) and easily implemented in a couple of lines of code.
Logistic Regression
No, Logistic Regression isn’t for regression problems. It’s actually for classification tasks. The algorithm applies a logistic function to a combination of features that predicts the outcome of a dependent variable. Of course, it wouldn‘t be true to the name if the independent variable wasn’t based on already predicted input variables. It’s split up into three categories:
Hire expert developers for your next project
- Binary Logistic Regression
- Multi-nominal Logistic Regression
- Ordinal Logistic Regression
Binary Logistic Regression is most commonly used when there are two possible binary classifictions or outcomes (yes or no; pass or fail). This can help in ways such as predicting if a student is likely to pass or fail a course or predicting if a tumor is cancerous or not. Multi-nominal Logistic Regression has three or more outcomes with no order, and Ordinal Logistic Regression has three or more outcomes with a natural ordering.
Developing a Machine Learning algorithm: What skills do you in your team?
Now that you have clarity about the popular ML algorithms, you are likely thinking about how to develop one for your mobile or web application.
The key question that probably comes to your mind is – What skills do I need in my team? What skills should I seek in my developers i.e. Java, JavaScript, Agile, Scrum, API development, experience working with IBM or AWS AI systems, etc?
At the time of writing this guide, Python is the most popular language for ML development. Python is simple, and that contributes greatly to its popularity.
Compared to several other languages, you need a shorter time to develop ML code in Python. This popular language has many libraries that make programming easier. Take the example of Pybrain, Scikit-learn, and XGBoost, which are libraries for ML coding using Python. Read more about the popularity of Python in “Top 5 best programming languages for Artificial Intelligence field”.
Wondering how to find competent JavaScript and Python developers and project managers? DevTeam.Space developers and dev teams have helped companies to build a number of ML and AI software products. Simply tell us your product specifications via this link and one of our account managers will get in touch.
Frequently Asked Questions
Bootstrap aggregating or bagging is a machine learning meta-algorithm that is designed to improve the accuracy of ML algorithms used in statistical classification.
Binary classification is the classification of data into two different groups.
AI or artificial intelligence is currently a stage of computer intelligence that has not yet been reached. Current systems are ML or machine learning systems that are able to cope with a narrow set of interpretations. AI will arrive when computers are able to learn new tasks and skills independently of humans.
These are formulas that allow computer programs to identify trends or patterns in data pools and cross-reference the results with past results to improve future results.
Regression algorithms are part of the Supervised Machine Learning algorithms family. Regression algorithms are used to predict the output values from pools of data.
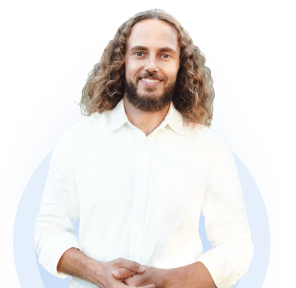
Alexey Semeney
Founder of DevTeam.Space
Hire Alexey and His Team
To Build a Great Product
Alexey is the founder of DevTeam.Space. He is award nominee among TOP 26 mentors of FI's 'Global Startup Mentor Awards'.
Hire Expert Developers