- Developers
- Developer Blog
- AI Software Development
- How To Build A Machine Learning Filing System To Classify Books
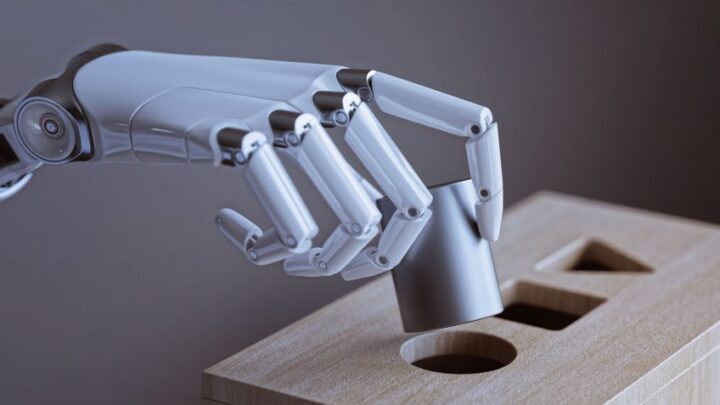
profile

By Aran Davies
Verified Expert
8 years of experience
Aran Davies is a full-stack software development engineer and tech writer with experience in Web and Mobile technologies. He is a tech nomad and has seen it all.
The steps you need to take to build a machine learning filing system to classify books are as follows:
1. Agree on a project scope
As the first step, you need to induct a competent project manager (PM) in your team and work with various stakeholders to define the project scope. You also need an IT architect and business analysts.
Together, they should work to define the project scope. I recommend that you start with building a web app with ML-powered document classification as its key functionality.
2. Choose an appropriate methodology for the project
It‘s now time to strategize the project. You need to choose the right methodology for this project, and I recommend the Agile methodology. Experts contend that the deployment of AI and ML systems benefit from the Agile methodology, as you can read in “5 ways to improve AI/ML deployments”.
3. Project planning and estimation
A detailed project plan is a key to the success of your project, moreover, you also need a budget-quality estimate for the project. The business stakeholders in your organization need these to provide the necessary green signals for the project.
Your PM and architect can consult our guides for this, e.g., they can read “AI development life cycle: Explained” to get a good grasp of the AI development lifecycle. You can also consult “How much does it cost to develop an AI solution for your company?”, which will help you with the project’s cost estimation.
4. Determine a development approach for the project
Your project team should adopt the following approach for this project:
- Use a managed cloud service so that you can focus on development, and not IT infrastructure management.
- Expedite the project with an NLP software development kit (SDK) or application programming interface (API).
- Utilize a test automation aid to enhance test coverage.
You can read “What is the best development approach to guarantee the success of your app?” to understand why this approach is useful.
5. Build your project team
You now need to induct the remaining roles for your project team, and these are as follows:
- UI designers;
- ML/AI developers with Python skills;
- Web developers with Node.js skills;
- Testers;
- DevOps engineers.
ML is a niche skill, therefore, you can expect this project to be a complex one. I recommend that you hire a field expert development team for such projects, as I have explained in “Freelance Development Teams vs Dedicated Development Teams: A Review”.
6. Use a managed cloud services platform
You can expedite the development of the proposed web app with NLP capabilities by using a managed cloud services platform. I recommend that you use a Platform-as-a-Service (PaaS) platform since you can get several advantages:
Hire expert developers for your next project
- Reputed PaaS providers manage cloud infrastructure, networking, storage, operating system, middleware, and runtime environment. This frees you up, therefore, you can focus on development.
- You can easily scale your web app when using a reputed PaaS platform since they provide robust application performance monitoring (APM) and auto-scaling solutions.
- It‘s easy to integrate database and 3rd party APIs when you use a PaaS platform.
- Well-known PaaS providers have robust DevOps tools, therefore, you can take advantage of continuous integration (CI) and continuous delivery (CD) capabilities.
You can read “10 top PaaS providers” to learn more about the advantages of using a PaaS platform.
AWS has excellent cloud capabilities, and it offers AWS Elastic Beanstalk, i.e., its PaaS platform. I recommend that you use it in this project.
7. Find an NLP SDK/API solution
Using an SDK/API solution for implementing the NLP capabilities could expedite your project, and I recommend that you use Amazon Comprehend. This is an NLP service from AWS, and it uses ML to find insights and relationships in texts.
Amazon Comprehend has several valuable features that will help you to build an ML filing system to classify books, e.g.:
- Keyphrase extraction;
- Sentiment analysis;
- Syntax analysis;
- Entity recognition;
- Relationship extraction;
- Custom entities;
- Language detection;
- Custom classification;
- Topic modeling;
- Multiple language support.
Read more about these in “Amazon Comprehend features”.
There is extensive documentation for Amazon Comprehend, e.g.:
- Amazon Comprehend developer guide;
- SDK documentation.
There are Amazon Comprehend SDKs in all popular languages, e.g., Java, Python, PHP, JavaScript, Ruby, .Net, and Go. You can also access videos that explain how to use Amazon Comprehend.
Visit “Amazon Comprehend developer resources” to access all of this documentation, moreover, you can install the SDK of your choice from here.
If you have more questions, you can check out the “Amazon Comprehend FAQs”. The pricing for Amazon Comprehend depends on the features used and resource consumption, and you can view “Amazon Comprehend pricing” for more information.
8. Sign-up for a test automation aid
The proposed web app should work with all browsers, therefore, you need to test it against different browsers and multiple versions of them.
It‘s not easy with an open-source test automation framework, however, Digital.ai provides a robust solution for this. You can use the mobile device & browser lab from Digital.ai, which offers a wide range of browsers.
Test reports and analytics are important for effective testing. Digital.ai offers Digital test analytics, which offers excellent test reports and analytics.I recommend that you use it.
9. Use an effective project management tool
I recommend that you use the scrum technique to manage this project since it‘s a proven technique to manage Agile projects. You should build scrum teams. These are small, cross-functional teams where developers and testers work together.
Your PM should perform the scrum master role, and the team should work on sprints, i.e., iterations. There are various activities for effectively managing a scrum team, e.g., sprint planning, daily stand-up meetings, sprint review meetings, and sprint retrospective meetings.
You can read more about scrum in “How to build a scrum development team?”. I recommend that you use a robust PM tool to manage this project. Asana is a good choice.
10. Developing the web app
Use JavaScript to develop the front-end of the web app. The open-source programming language is versatile, and it has a wide range of frameworks and libraries.
You can develop the front-end using JavaScript, HTML, and CSS. Alternatively, you can use popular open-source frameworks like Angular or React.js.
Node.js is a great choice to develop the back-end for the web app. This open-source runtime environment facilitates creating performant and scalable web apps, and it has a vibrant developer community. I recommend you use it for back-end web development.
You can use a popular IDE (Integrated Development Environment) like Eclipse to code the app. IntelliJ IDEA is another well-known IDE.
Hire expert developers for your next project
1,200 top developers
us since 2016
Developing this web app requires the following steps:
- Design a user-friendly UI.
- Integrate Amazon Comprehend SDK in your app.
- Test the app, and deploy it. You can read “Deploying Node.js applications to AWS Elastic Beanstalk” for guidance.
A few useful tips while building a machine learning filing system
Consider the following tips:
1. Understand the wide range of potential of machine learning techniques
Machine learning techniques can be powerful. Take the example of data analysis in the financial services industry.
Investors routinely read 10-K SEC filings to understand the status and worth of companies. A team of researchers led by Tiffany Jiang conducted an experiment.
They examined whether machine learning models can derive useful insights from 10-K SEC filings. Researchers came up with a machine learning model, which delivered 85% accuracy. E.g., their ML algorithms analyzed financial information to predict the likelihood of mergers.
That’s just one example. If you look at the financial markets, you find “big data” (high-dimensional data) everywhere. The question is how to gain valuable insights from large datasets containing financial information.
Companies in the financial services industry can use data science and ML systems to gain insights from financial statements. This helps investors and market participants to understand how companies are performing, e.g., they can get alerts about poor performance.
Other kinds of companies in other sectors can also gain valuable insights from big data using machine learning. They can use different approaches for this, which are as follows:
- Supervised machine learning;
- Unsupervised machine learning;
- Semi-supervised machine learning;
- Reinforced machine learning.
2. Study how ML systems can work with different forms of data
In your organization, you have data available in two ways. Data stored in Excel files or other database tables are structured data. Texts, web pages, comments, video files, audio files, etc. are unstructured data.
Businesses find it easier to gather insights from structured data. However, gaining insights from unstructured data can be hard. ML algorithms and NLP (Natural Language Processing) systems can help organizations to gain insights from unstructured data.
3. Keep the training data of your ML systems secure
The success of your machine learning system depends on the quality of the training data. Hackers often try to corrupt this data. E.g., they might insert wrong information. This is called “data poisoning”.
If you have trained models with such corrupted datasets, then the models will make wrong decisions. The consequences can be damaging. Watch out for such attacks. Remember that getting new data for training your ML models can be expensive, therefore, proactively secure your large datasets.
4. Plan for computational resources if you need to use deep learning
Do you plan to use deep learning in your project? It’s a subset of machine learning, however, there are key differences.
Deep learning uses a highly sophisticated type of machine learning. It uses “deep neural networks”, which are modeled after the human brain.
You need to feed very large datasets to deep learning systems. However, such systems deliver results quickly. You don’t need any significant human intervention either.
Remember that deep learning requires significantly higher computational resources than machine learning. This includes more powerful hardware. You will need to use GPUs, therefore, you should plan accordingly.
5. Use established algorithms to implement predictive modeling
Do you plan to use predictive modeling in your project? I recommend you use well-established algorithms. The following are a few examples:
- “Random Forest”;
- “Generalized Linear Model (GLM) for two values”;
- “Gradient Boosted Model”;
- “K-Means”;
- “Prophet”.
6. Pay attention to the relevant metadata
You will likely need multiple iterations before perfecting your machine learning model. In this process, you will need to compare the latest model with the earlier models. You can compare them meaningfully only if you save the relevant metadata from the earlier iterations. Collect the following types of metadata:
- Data;
- Model;
- Model type;
- Steps in feature preprocessing.
7. Familiarize yourself with the important Python machine learning libraries
Software engineers use Python in machine learning projects due to many reasons. A few of them are as follows:
Hire expert developers for your next project
- Programmers can focus on simplicity while using Python. Machine learning projects can be complex. Therefore, a programming language that encourages simplicity is a great asset in such projects.
- Python is easy to learn.
- Code written in Python is easy to read.
- You can build prototypes quickly using Python.
There’s yet another important reason for you to use Python in a machine learning project. You can use excellent Python libraries for developing ML systems. The following are a few examples:
- Numpy;
- SciPy;
- Scikit-learn;
- Theano;
- TensorFlow;
- Keras;
- PyTorch;
- Pandas;
- Matplotlib.
Your software development team should be familar with them.
8. Focus on hiring skilled, experienced, and motivated developers
I talked about hiring developers from the right company when developing an ML application. I want to stress hiring the right developers too. ML projects tend to be complex, therefore, you need to focus on skills, experience, and competencies.
When you hire developers, look for in-depth Python skills. You should expect a good knowledge of Python ML libraries. The candidates should demonstrate a thorough understanding of ML algorithms, and they should be familiar with different ML platforms.
Don’t focus on technical questions alone. Try to assess the relevant experience in ML projects. Ask candidates how they solved various complex problems, and assess their problem-solving skills.
You should expect them to have the following competencies:
- The ability to see the perspective of end-users;
- Communication skills;
- Passion for excellent;
- Commitment to your project objectives;
- Collaboration skills;
- Teamwork.
9. Pay attention to code review and testing
I hardly need to explain the importance of testing. Most organizations pay close attention to validation activities like testing.
However, verification activities like code review can sometimes fall through the cracks. Stringent deadlines and the lack of experienced reviewers often compel organizations to cut corners as far as code review is concerned.
I recommend you plan adequately so that you can have structured code reviews in your ML project. Remember that you need experienced reviewers. ML is a niche area, therefore, it’s not easy to find such reviewers. You can engage DevTeam.Space for code review.
You need to incorporate code review proactively in your project plan. This will help you to identify defects earlier.
Planning to launch a machine learning filing system to classify books, etc.?
A machine learning filing system to classify documents will certainly add significant value to your organization. This guide, platforms, tools, frameworks, and SDKs can expedite the project, however, it‘s still a complex project.
You should engage a reputed software development company for such projects. Our guide “How to find the best software development company?” can help you to find such a development partner.
Reach out to DevTeam.Space if you need help. A dedicated account manager will explain how we can assist in developing a market-competitive machine learning filing system.
Frequently Asked Questions on Machine Learning Filing Solution
ML stands for machine learning. Machine learning involves computer programs undertaking tasks such as recommending movies etc., the results of which they are able to learn in order to improve future predictions.
ML is an ideal technology for filing systems as it allows them to gain in accuracy the more that they are used. If used enough, ML systems will eventually be able to improve to such an extent as to make the filing system almost flawless.
If you are looking for expert ML developers then head to DevTeam.Space. The platform has years of experience developing complex machine learning solutions.
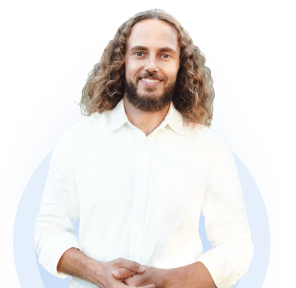
Alexey Semeney
Founder of DevTeam.Space
Hire Alexey and His Team
To Build a Great Product
Alexey is the founder of DevTeam.Space. He is award nominee among TOP 26 mentors of FI's 'Global Startup Mentor Awards'.
Hire Expert Developers