- Developers
- Developer Blog
- Software Development
- How to Create an AI Solution?
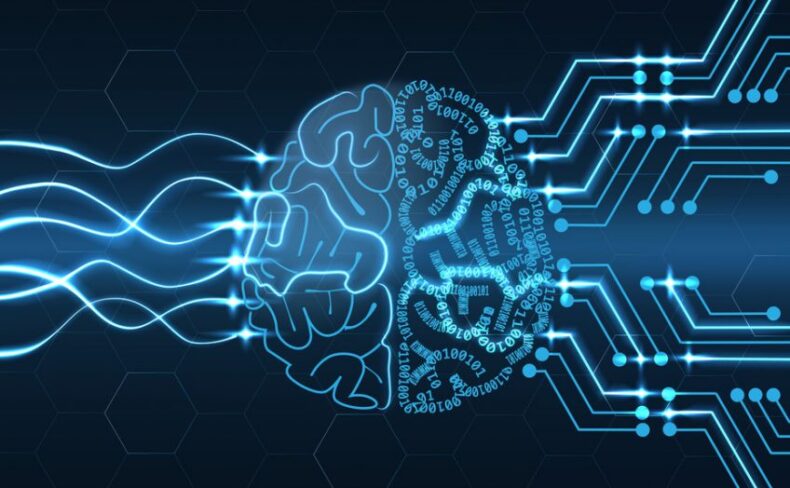
profile
Verified Expert
1 year of experience
Jamie is a dedicated, personable, and enthusiastic Microsoft MVP in Artificial Intelligence and Certified Technical Specialist. He writes deeply technical articles that combine a strong academic background with extensive industry experience in software and application development.
Wondering how to create an AI solution?
AI is set to become one of the leading software development growth markets. According to Statista, the global market for AI is expected to reach $126 billion by 2025.
Developing an AI system requires a deep understanding of supervised and unsupervised machine learning algorithms, Python programming language, ML development frameworks, etc.
If you don’t have a professional team with relevant expertise to take on the task of AI development, then submit a request for a complimentary discovery call, and one of our tech account managers who managed similar projects will contact you shortly.

Before we get into what‘s involved in building AI systems that leverage artificial intelligence and data science, let‘s start with a definition of what the term “artificial intelligence” means:
Artificial intelligence (AI) is intelligence exhibited by machines. In computer science, the field of AI research defines itself as the study of “intelligent agents”: any device that perceives its environment and takes actions that maximize its chance of success at some goal.
Colloquially, the term “artificial intelligence” is applied when a machine mimics “cognitive” functions that humans associate with other human brain functions such as “learning” and “problem-solving”.
How to Create AI Software?
If you‘re thinking of exploring artificial intelligence or machine learning, you need to ask yourself the question:
Do I really need to implement artificial intelligence in my software solution?
This raises another question:
How do you know if you need to create an AI solution!?
Here are some guidelines:
- Do you deal with vast quantities of data?
- Is the data in various formats?
- Are you dealing with frequently changing parameters?
- Is the data arriving at ever-increasing velocity?
If you have answered yes to most of these points, then you might want to consider integrating AI and machine learning into your tech stack.
Get a complimentary discovery call and a free ballpark estimate for your project
Trusted by 100x of startups and companies like
In order to have a better insight into this topic, check out the pros and cons of artificial intelligence.
An Example: Managing Email Spam
Consider an email spam problem and consider the problems you‘d encounter by implementing the application using traditional programming techniques. Well, most of this can be alleviated by implementing an AI/ML approach.
The problem
Email spam detection is ultimately a text classification problem.
Fortunately, this is a well-studied area, and several techniques can be employed by the machine to determine to which Category the new incoming email belongs.
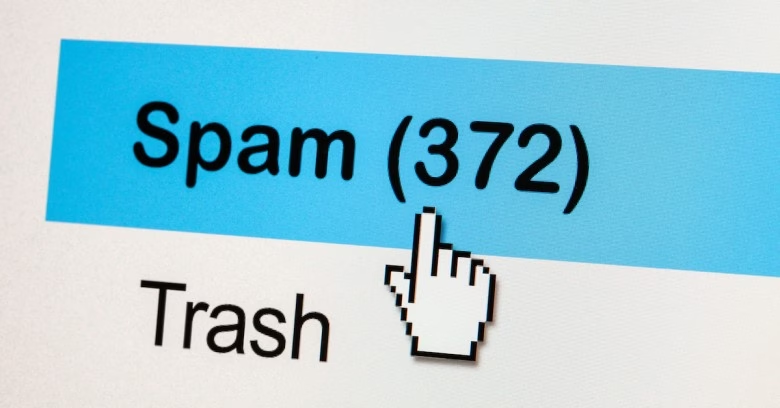
Algorithm and approach
Before we delve into the technicalities of which API to use when you create AI software, it can be helpful to further understand the background of how such a solution can be approached by using Supervised Learning.
If you‘re unfamiliar with this, Supervised Learning is a technique that involves the construction of a “Classifier”. The Classifier neural networks are responsible for categorizing text into a Category (sometimes known as a Label).
Some classification techniques include:
- Naïve Bayes;
- Maximum Entropy;
- Support Vector Machines (SVM).
Naïve Bayes is a relatively simple and accurate way for the machine to identify which category an email belongs to.
Bayesian Theorem is a probability theory used to arrive at predictions considering recent evidence. The theorem was discovered by an English Presbyterian and mathematician called Thomas Bayes and published posthumously in 1763.
The rule is written like this:
p(A|B) = p(B|A) p(A) / p(B)
To deconstruct the rule, here are descriptions of each component that form it:
p(A|B)
‘The probability of A given B’. This basically means the probability of finding observation A, given that some part of evidence B is there. This is what we want to find out.
p(B|A)
This is the probability of the evidence turning up, given the outcome obtained.
p(A)
This is the probability of the outcome occurring, without the knowledge of the new evidence.
p(B)
This is the probability of the evidence arising, without regard to the outcome.
We can apply this rule to our email spam problem and plug in the parameters:
P(spam |words) = P(words/spam)P(spam) / P(words)

This is all fine and well but it may be hard to visualize without data and values. So, taking the above into consideration, imagine we had a database of 100 emails.
We also believe that emails that contain the word “buy” are spam emails. We can now apply the Bayes Rule:
Training Data
100 emails in total
60 of those 100 emails are spam
48 of those 60 emails that are spam have the word “buy”
12 of those 60 emails that are spam don’t have the word “buy”
40 of those 100 emails aren’t spam
4 of those 40 emails that aren’t spam have the word “buy”
36 of those 40 emails that aren’t spam don’t have the word “buy”
Hire expert software developers for your next project
1,200 top developers
us since 2016
What is the probability that an email is a spam if it has the word “buy” in the content?
The answer to the above is as follows: There are 48 emails that are spam and have the word “buy” and there are 52 emails that have the word “buy”: 48 that are spam plus 4 that aren’t spam.
So the probability that an email is spam if it has the word “buy” is 48/52 = 0.92
As mentioned previously, the rule and notation are based on probabilities, so we can redefine the problem to use probabilities rather than quantities. Using the same database of emails.
60% of those emails are spam.
80% of those emails that are spam have the word “buy”.
20% of those emails that are spam don’t have the word “buy”.
40% of those emails aren’t spam.
10% of those emails that aren’t spam have the word “buy”.
90% of those emails that aren’t spam don’t have the word “buy”.
What is the probability that an email is spam if it has the word “buy”? The notation to arrive at the answer looks like this:
P(spam) = the probability that an email is a spam.
P(not spam) = the probability that an email isn’t spam.
P(“buy”|spam) = the probability that an email that is spam has the word “buy”.
P(“buy”|not spam) = the probability that an email that isn’t spam has the word “buy”.
P(spam|”buy”) = the probability that an email that has the word “buy” is spam.
So P(spam|”buy”) is the answer we are looking for
P(“buy”|spam) * P(spam) counts all the emails that are spam and have the word “buy”.
P(“buy”|not spam) * P(not spam) counts all the emails that aren’t spam and have the word “buy”.
Summing the previous two P(“buy”|spam) * P(spam) + P(“buy”|not spam) * P(not spam) we count all the emails that have the word “buy”.
Meaning the resulting equation looks like this: (This is Bayesian Theorem)
P(spam|”buy”) = P(“buy”|spam) * P(spam) / (P(“buy”|spam) * P(spam) + P(“buy”|not spam) * P(not spam))
Or , to inject the numbers: 0.8 * 0.6 / (0.80.6 + 0.10.4) = 0.48 / 0.52
The result of the simulation was: 0.922248596074798
In Plain English
After running incoming emails through this classification model, we can safely assume that with a probability of 92%, if emails contain the word “buy”, they should be placed in the spam folder!
Training Data
The neural network classifier must be “trained” with sample datasets prior to determining future text classifications. In our email example, we had 100 emails, some contained the word “buy” and others didn‘t.
Without an accurate training process, the machine cannot accurately make reliable predictions about future events using data.
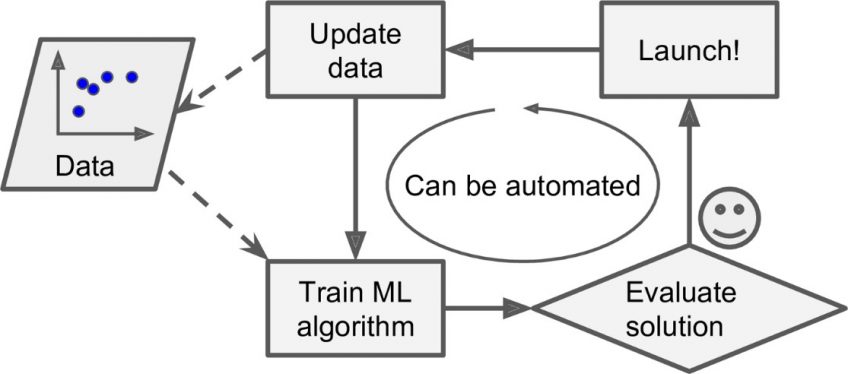
APIs
With the theory and example out of the way, you now have an appreciation for how probabilities can be determined by the machine.
Rather than code up numerous combinations of string patterns and complex business rules, spam identification can be determined using maths. This is a use case in which the machine will excel in!
The great news is that you don‘t need to code up theorems such as the Bayes Rule when you create AI software, the heavy lifting is now being done for you by the likes of Microsoft and IBM!
Hire expert software developers for your next project
You can create an account on Microsoft Azure and using a graphical user interface leverage artificial intelligence APIs that get exposed as REST endpoints. You simply pass in the parameters and the APIs return JSON with your results.
For example, a cloud computing service provider, Azure offers a Topic Detection API. This returns the detected topics for a list of submitted text records. A topic is identified with a key phrase, which can be one or more related words.
This is ideal for mining short, human-written text such as reviews and user feedback. Introducing an API like this into your stack could help your business gain actionable insights from existing datasets.
You can see an example of the Topic Detection API in action in the following screenshot:
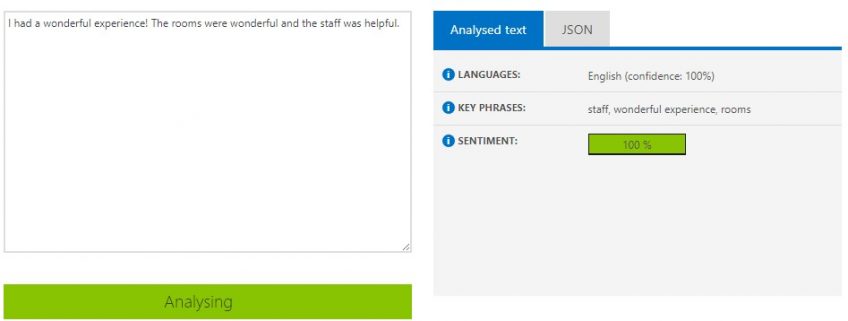
Note how the API has detected the Language, Key Topics, and Sentiment. In this screenshot, you can see the underlying JSON:
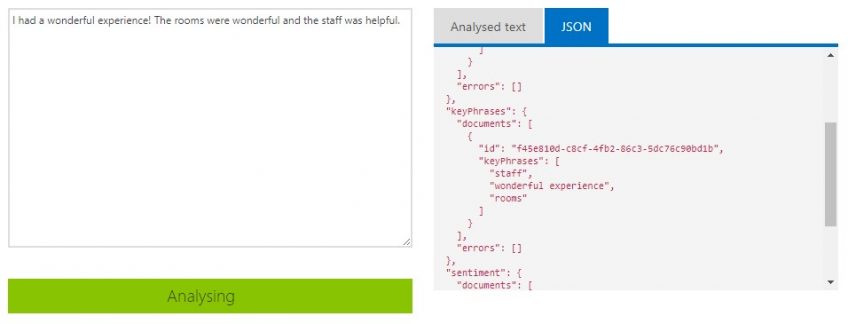
Imagine you had to manually code classes and custom APIs to determine these data points. Whilst these APIs do attract a cost, the tricky thinking has already been done, thereby freeing you up to solve the business problem.
Additional Applications of Artificial Intelligence
We‘ve covered email classification and explained how one can mine relevant data to glean actionable insights, other potential areas where AI is starting to be applied include:
AdTech and Sentiment Analysis
The UK start-up SocialOpinion leverages artificial intelligence to identify the current sentiment of brands, products, and services on social media. In addition to this, Microsoft Cognitive Services LUIS is also used to try and determine sales leads.
Crime Prediction
The company Pred Pol built a software product that leverages big data, machine learning algorithms, and analytics. The vision was to see if the software could use historical data sets to anticipate crime locations and times and allow officers to pre-emptively prevent these crimes from occurring.
The software used the following to help achieve this:
- Past type of crime;
- Crime location;
- Date/time of the crime.
Research has shown that additional crimes tend to occur close to the original crime spot. At the start of each shift, officers examine Google Maps which are overlaid with boxes that indicate potential criminal hotspots.
A data scientist working on this AI operation to reduce crimes should establish model accuracy by setting a minimum acceptable threshold. Provided the metrics are right, the model accuracy should dramatically help to reduce crime.
Driverless Cars
NVidia, a firm over two decades old with a history in computer graphics has partnered with leading names in the automotive industry, including Audi, Mercedes – Benz, etc., to build the next generation of autonomous vehicles powered by the NVIDIA DRIVE AGX platform.
This computer vision technology can process large volumes of sensor data and understand in real-time what’s happening around the vehicle, the location of itself on a map, and plan safe paths ahead.
The Future of Artificial Intelligence
At the time of writing, Facebook developed an AI system that created its own language. The system had developed a more refined vocabulary to communicate with itself. Programmers at Facebook shut down the system when they realized it was no longer communicating in readable English.
The system was originally trained in English but soon diverged from this when it realized there were more efficient ways to communicate with each “AI agent”. Matrix anyone?!
Ready to Create an AI System?
We‘ve covered a lot in this blog post, from the origins of AI, to how you can create an AI system, as well as some of the current APIs out there that shield you from developing AI systems with complex deep learning algorithms and AI model training tasks that are often involved in an AI project.
As much as we‘d like to be able to, it can be hard to predict the future of artificial intelligence. Cognitive computing is an evolving space, if only we had AI for the AI.
If you are developing an AI app and need to scale your team with additional skills and expertise then take a moment to tell us about your project requirements here.
One of our dedicated tech account managers will be in touch to show you similar projects we have done before and share how we can help you create your own AI system.
Frequently Asked Questions on How to Create an AI System
AI models, if trained on high-quality data by data scientists, can offer the following benefits:
• Reduce human errors;
• AI solutions systems work 24×7;
• AI-powered digital assistants help with common day-to-day tasks;
• Systems using AI make decisions based on data and evidence for a particular task;
• AI offers intelligent automation.
An example is the growing use of AI-powered tools for cybersecurity by software engineers. Cyber-attacks are increasingly getting sophisticated, and data breaches put the sensitive personal information of many people at risk. Organizations can create an AI system to help fight cybercriminals by analyzing massive volumes of structured data and unstructured data to detect potential suspicious behavior online.
Unfortunately, authoritarian states are using AI technology to conduct intrusive surveillance. AI capabilities like facial recognition using natural language processing have become useful tools in the hands of these states to suppress and marginalize minorities.
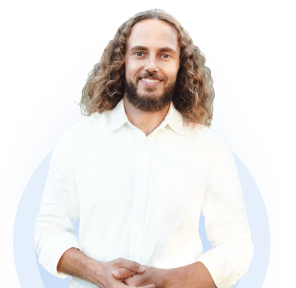
Alexey Semeney
Founder of DevTeam.Space
Hire Alexey and His Team To Build a Great Product
Alexey is the founder of DevTeam.Space. He is award nominee among TOP 26 mentors of FI's 'Global Startup Mentor Awards'.
Alexey is Expert Startup Review Panel member and advices the oldest angel investment group in Silicon Valley on products investment deals.