- Developers
- Developer Blog
- AI Software Development
- How to Make a Speech Recognition System
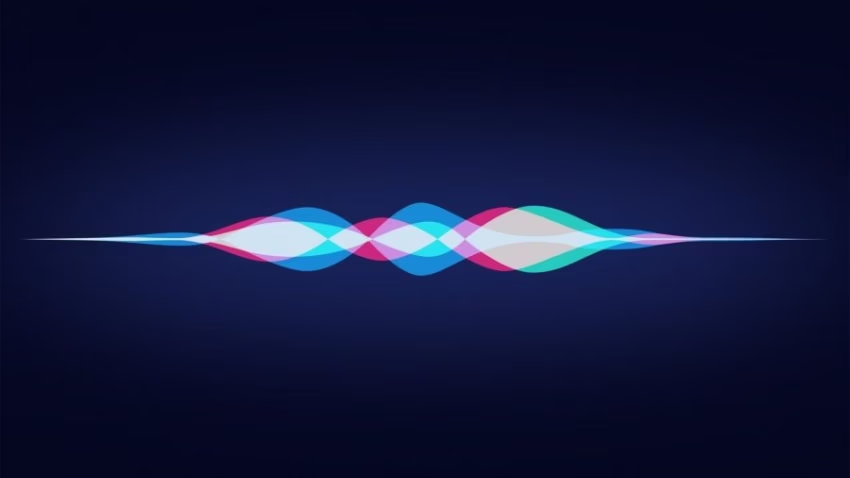
profile

By Sam Palmer
Verified Expert
1 year of experience
Sam is a professional writer with a focus on software and project management. He has been writing on software-related topics and building PHP based websites for the past 12 years.
Looking to make a speech recognition system? In this guide, I’ll explain how to do it step by step. Keep reading!
In this article
- Tools to Use for Building a Speech Recognition System
- Finding Developers Who Can Help Build a Speech Recognition System
- Key Considerations While Implementing the Speech Recognition Technology
- Frequently Asked Questions on How to Make a Speech Recognition System
The speech recognition market size is projected to reach $7.14 billion in 2024 globally. The United States’ speech recognition market value will total $1,903.00 million in 2024. This lucrative market is expected to grow at a CAGR of 14.24% between 2024 and 2030, reaching $15.87 billion by 2030.
Before we jump into how to make a speech recognition system, let’s take a look at some of the tools you can use to do it.
Tools to Use for Building a Speech Recognition System
Commercial APIs
Many of the big cloud providers have APIs you can use for voice recognition. All you need to do is query the API with audio in your code, and it will return the text. Some of the main ones include:
- Google Speech API
- Microsoft Cognitive Services — Bing Speech API
- Amazon Alexa Voice Service
- Facebook’s Wit.ai
This is an easy and powerful method, as you’ll essentially have access to all the resources and speech recognition algorithms of these big companies.
Of course, the downside is that most of them aren’t free. And, you can’t customize them very much, as all the processing is done on a remote server. For a free, custom voice recognition system, you’ll need to use a different set of tools.
Open Source Voice Recognition Libraries
To build your custom solution that recognizes audio and voice signals, there are some really great libraries you can use. They are fast, accurate, and free. Here are some of the best available — I’ve chosen a few that use different techniques and programming languages.
CMU Sphinx
Get a complimentary discovery call and a free ballpark estimate for your project
Trusted by 100x of startups and companies like

CMU Sphinx is a group of recognition systems developed at Carnegie Mellon University – each designed for different purposes. It is written in Java, but there are bindings available for many languages. This means you can use the libraries and voice recognition methods even if you want to program in C# or Python. There are some great components you need to develop a voice recognition system.
For an awesome example of an application built using CMU Sphinx, check out the Jasper Project on GitHub.
KALDI
Kaldi, released in 2011 is a relatively new toolkit that’s gained a reputation for being easy to use. It uses the C++ programming language.
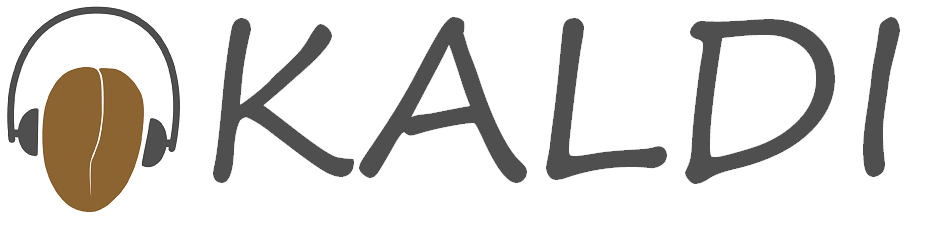
HTK
HTK, also called the Hidden Markov Model Toolkit, is made for the statistical analysis modeling techniques. It’s owned by Microsoft, but they are happy for you to use and change the source code. It uses the C programming language.
Where to Get Started?
If you’re new to building this kind of system, I would suggest you to go with something based on Python that uses the CMU Sphinx library. Check out this quick tutorial that sets up a very basic system in just 29 lines of Python code.
Finding Developers Who Can Help Build a Speech Recognition System
Needless to say, speech recognition programming is an art form, and putting all this together is a heck of a job. To create something that really works, you’ll need to be a pro yourself or get some professional help. Learn how to build an agile development team and why it’s important for the success of your app.
Software teams at DevTeam.Space build these kinds of systems all the time and can certainly help you get your app to understand your users very quickly.

Key Considerations While Implementing the Speech Recognition Technology
Keep the following key questions and considerations in mind when you create and implement speech recognition software:
Hire expert developers for your next project
1,200 top developers
us since 2016
1. Define your business problems or opportunities to find the right use case
By now, you know that building a speech recognition system involves complexities. You need to first analyze your business problems and opportunities. Assess whether you have a viable use case for using the speech recognition technology.
Speech recognition technology has given rise to applications facilitating voice search and recognizing speech signal. Digital assistants like Apple’s Siri accept voice commands from users and respond to their requests.
Many sectors like healthcare, government, etc. have high-value use cases involving this promising technology, and your organization might have one too. Identify the right use case.
2. Decide the functionality and features to offer
A user of an Apple iPhone has certain specific needs when using Apple’s Siri. Similarly, Google Home and other popular automatic speech recognition software deliver tangible value to users. These organizations undertook large-scale studies to determine the scope of their “Artificial Intelligence” (AI) projects.
They often pushed the boundary and offered very helpful features. E.g., “Apple Dictation” is a useful speech-to-text app for Apple devices. Another example is the “Voice Access” app from Google. It helps users to make phone calls in hands-free mode.
You need to study your business requirements carefully. Subsequently, you need to decide the functionality and features to offer. Plan to support all key operating systems.
3. Plan the project meticulously
Plan meticulously so that you prepare sufficiently for the entire AI development lifecycle. Do the following:
- Define why you would use AI and what you will automate.
- Identify relevant data sources and gather large enough datasets consisting of various speech patterns to build a large vocabulary speech recognition solution.
- Determine the AI capabilities you need, e.g., “Deep Learning” (DL), “Natural Language Processing” (NLP), speech recognition, etc.
- Evaluate popular SDLC methodologies like Agile and choose a suitable methodology.
- Plan the relevant phases like requirements analysis, design, development, testing, deployment, and maintenance.
4. Decide the technical capabilities you will use, e.g., “Speech-to-text”
Depending on your business requirements, you need to choose one or more technical capabilities within the large landscape of AI. E.g., you might need to explore the following:
- “Machine Learning” (ML);
- “Deep Learning” (DL);
- NLP;
- Acoustic modeling for speech recognition;
- Generating optimal word sequences using “Automatic Speech Recognition” (ASR) systems;
- Using acoustic modeling for recognizing phonemes, which could help with speech recognition;
- “Hidden Markov Model” (HMM) decomposition, which helps to recognize speeches where there’s interference from another background speaker or background noise;
- Using continuous speech recognition;
- “Limited vocabulary” speech recognition techniques;
- Measuring speech recognition accuracy by using the “Word Error Rate” (WER);
5. Developing capabilities vs using 3rd party APIs
You will likely design and develop software to suit your requirements. For this, you will likely code algorithms and modules using Python. There are very good tutorials to create speech recognition software using Python, which will help.
In some scenarios, you might want to use market-leading APIs. This could save some time since you won’t reinvent the wheel. The following are a few examples of such APIs:
- The “Speech-to-text” API from Google Cloud: This API helps you to transcribe your speech data in real-time;
- The Automatic Speech Recognition (ASR) system from Nuance: Nuance offers an ASR system, which is especially helpful for customer self-service applications;
- IBM Watson “Speech to text” API: You can use to add capabilities to transcribe speech signals;
- “Speech Recognizers” like CMU Sphinx “Recognizer”.
Hire expert developers for your next project
Planning to Implement a Speech Recognition System?
Speech recognition tech is finally good enough to be useful. Pair that with the rise of mobile devices (and their annoyingly small keyboards), and it’s easy to see it taking off in a big way. To keep up with your competition and make your customers happy, why not learn how to make a voice recognition program and implement it into your products?
If you are looking for experienced software engineers to help you with the development of a speech recognition solution, DevTeam.Space can help you.
Get in touch via this quick form stating your initial requirements for speech recognition system project. One of our technical managers will get back to you and connect you with expert software developers experienced in developing market-competitive speech recognition platforms.
Frequently Asked Questions on How to Make a Speech Recognition System
It is a software system that is able to recognize what people are saying to it. Speech recognition systems vary from simple human speech recognition saying yes or no to sophisticated machine learning programs such as SIRI understanding spoken language using complex neural networks.
The process is simple. As the machine listens to the human voice it breaks down the sounds in such a way that it is able to recognize individual words. More sophisticated programs use machine learning to improve the accuracy of a speech recognition task. Such systems are able to learn accents, different pitches, tones of voice, etc.
Any program that requires machine learning will require a team of expert developers including voice recognition software. If you have such developers then they will be able to build a voice recognition technology for you. If you don’t, however, then you should onboard developers from an experienced software development platform such as DevTeam.Space to build next-level speech recognition applications.
Related AI Software Development Articles
Learn more about developing AI software from our expert articles:
- How to Interview and Hire AI Developers
- How Much Does Artificial Intelligence Cost to Develop?
- How To Create an AI SaaS Product
- How to Implement Predictive Analytics for Business Processes
- How to Hire Data Scientists
- How to Integrate AI into a Database
- How to Create an AI Chatbot for Customer Service
- How Applying AI in Manufacturing Will Save Billions of Dollars for Industry?
- 10 Real Life Examples of AI Use Cases
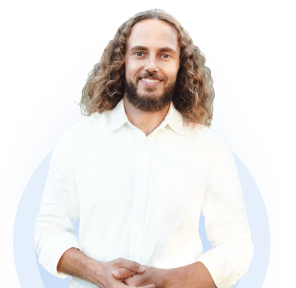
Alexey Semeney
Founder of DevTeam.Space
Hire Alexey and His Team To Build a Great Product
Alexey is the founder of DevTeam.Space. He is award nominee among TOP 26 mentors of FI's 'Global Startup Mentor Awards'.
Alexey is Expert Startup Review Panel member and advices the oldest angel investment group in Silicon Valley on products investment deals.